Projects
Forte: Composing Diverse NLP Tools for Text Retrieval, Analysis and Generation
Forte is a flexible composable system designed for text processing, providing integrated architecture support for a wide spectrum of tasks, from Information Retrieval to tasks in Natural Language Processing (including text analysis and language generation). Empowered by principled abstraction and design principles, Forte provides a platform to gather cutting-edge NLP and ML technologies in a composable manner.
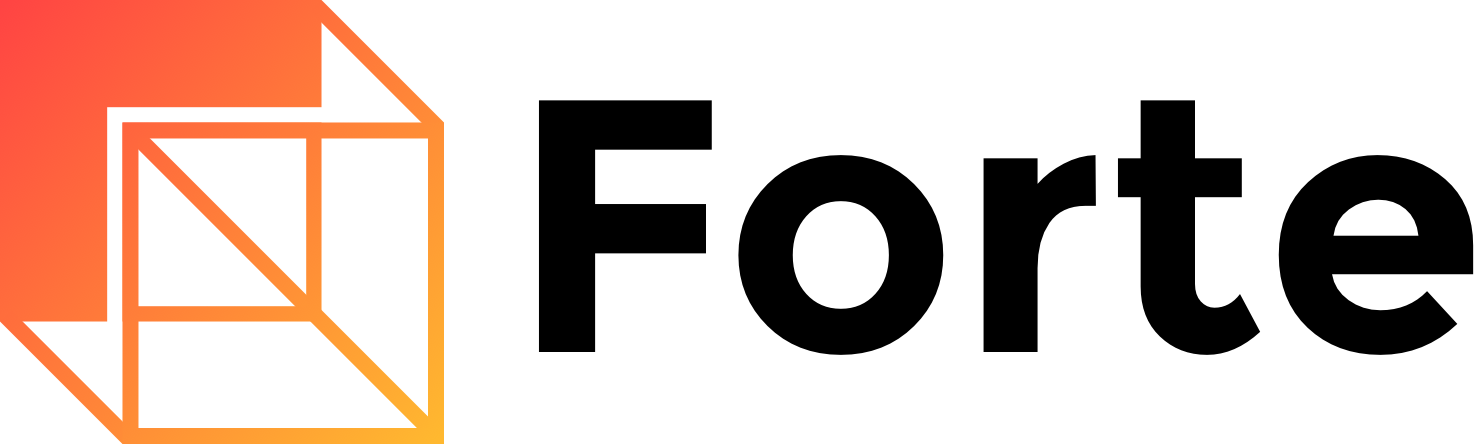
Texar: A Modularized, Versatile, and Extensible Toolkit for Text Generation
Texar-PyTorch is an open-source toolkit based on PyTorch, aiming to support a broad set of machine learning especially text generation tasks, such as machine translation, dialog, summarization, content manipulation, language modeling, and so on. Texar is designed for both researchers and practitioners for fast prototyping and experimentation.
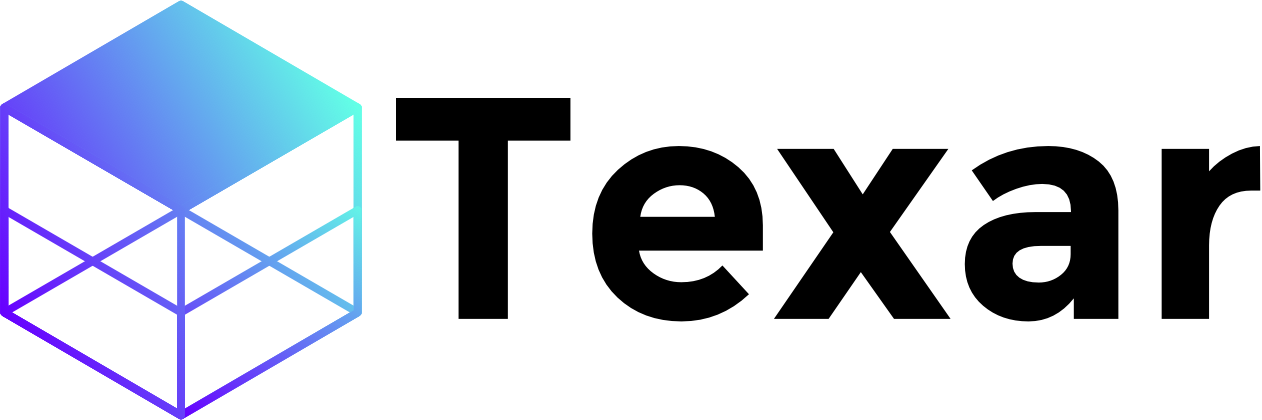
Online Algorithm for PMU Data Processing (OLAP)
We implemented OLAP by C# based on Project Alpha for the real-time application. Project Alpha is elite version of Open PDC. It provides a jump start to developing new products based on the Grid Solutions Framework’s Time-Series Library (TSL) technology complete with all needed components for standalone time-series processing application. The code developed on Project Alpha can be run on Open PDC as action adapter.
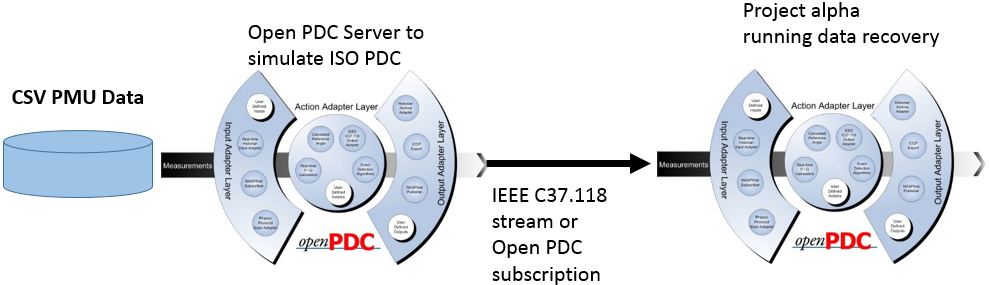
Publication: Pengzhi Gao, Meng Wang, Scott G. Ghiocel, Joe H. Chow, Bruce Fardanesh, and George Stefopoulos. Missing Data Recovery by Exploiting Low-dimensionality in Power Systems Synchrophasor Measurements. IEEE Trans. Power Systems, 2016, 31 (2): 1006-1013.
Patent: Meng Wang, Pengzhi Gao, and Joe H. Chow. “A low-rank-based missing PMU data recovery method.” Application No.: 62/445305, Filed January 12, 2017.
Neural Style Transfer
We implemented a neural-style algorithm [R1] that takes three images, a content image, a style image, and the input image you want to style. The key idea is to define two loss functions as follows. $L_{content}$ computes how different the content of two images are. $L_{style}$ computes the difference between two images in terms of their style. Then, we will transform the input image by minimizing the content and style losses with backpropagation and create an image that matches the content of the content image and the style of the style image.
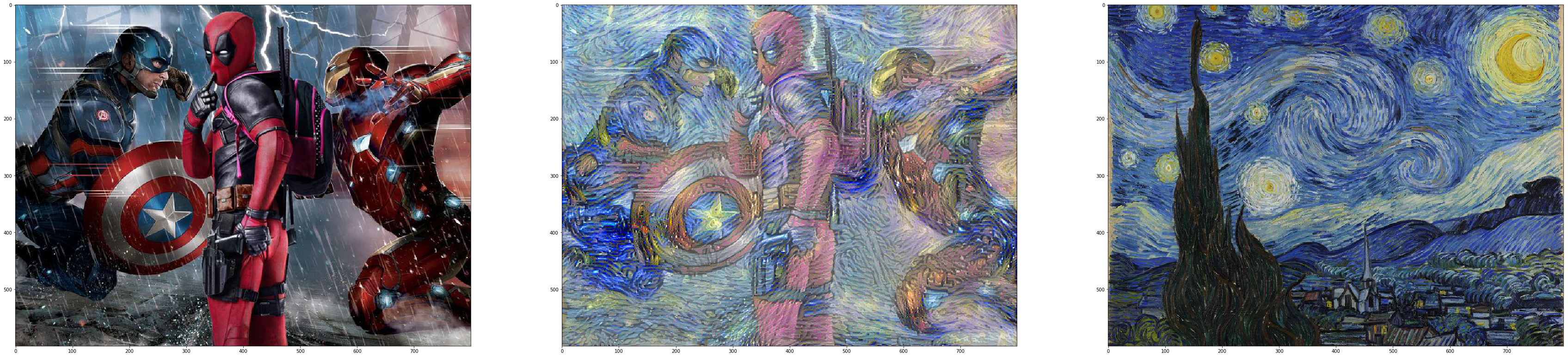
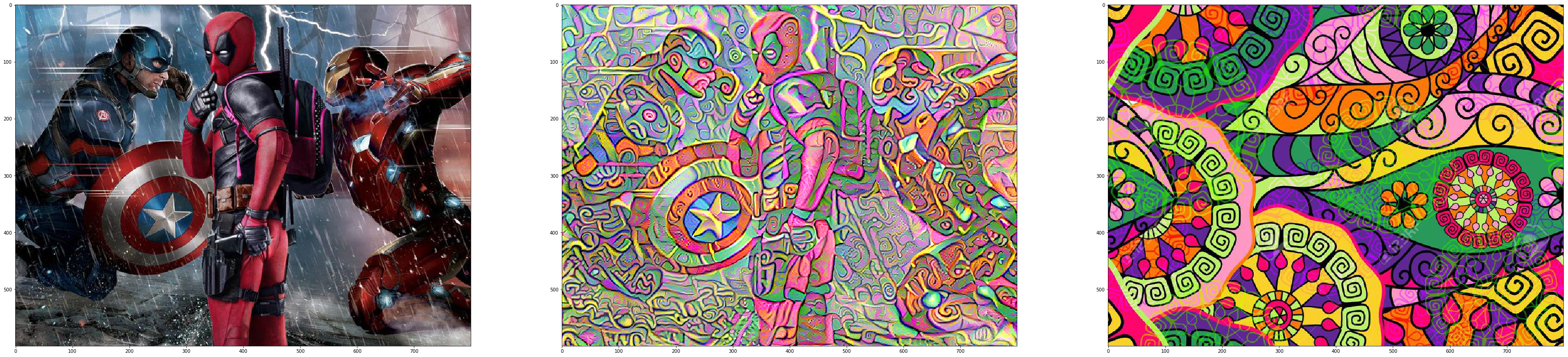
[R1] Leon A. Gatys, Alexander S. Ecker, Matthias Bethge. A Neural Algorithm of Artistic Style.